AI-Driven Service Delivery
Introduction
For the Global Design Futures Unit 2018 we are working on a project called " The Future of Government 2030+", a brief launched by the European Commission’s Joint Research Centre (JRC). We are working in collaboration with London Borough of Camden and Public Collaboration Lab to explore which could be the new relationships between citizens, businesses and government.
The society is continually changing the way people communicate and interact due to the rapid development of technology in the last several decades. Nowadays, the development of new technologies such as artificial intelligence has deeply affected how the government works and it may shape new models of service delivery.
This blogpost will briefly illustrate how we envision the service delivery in a future of total surveillance, contextualising the focus on the social isolation issue. It will also include the feedback we received from the recent workshop with professionals and how we plan to iterate the project in the further stage.
Our concept
Future Scenario- AlGo-vernment
Based on the scenario that we have chosen, in 2030, the government works based on AI automated decision making system. The boundary is blurred between tech company and government. Surveillance is strong and no privacy for citizens. In such circumstances, people are more and more isolated as they work and communicate mainly in the virtual space. Moreover, everyone should take charge of their own health condition to get healthcare, this society tends to become ‘Blaming culture’ . People have to involved within “social credit” system, an end-to-end social control where each citizen receives a numeric index of its trustworthiness and virtue.
Challenge
“What if the quality of resources used in the social care service delivery will depend on citizens’ efforts on prevention?”
Brief concept-
AI-driven model optimizing the usage of public service resources
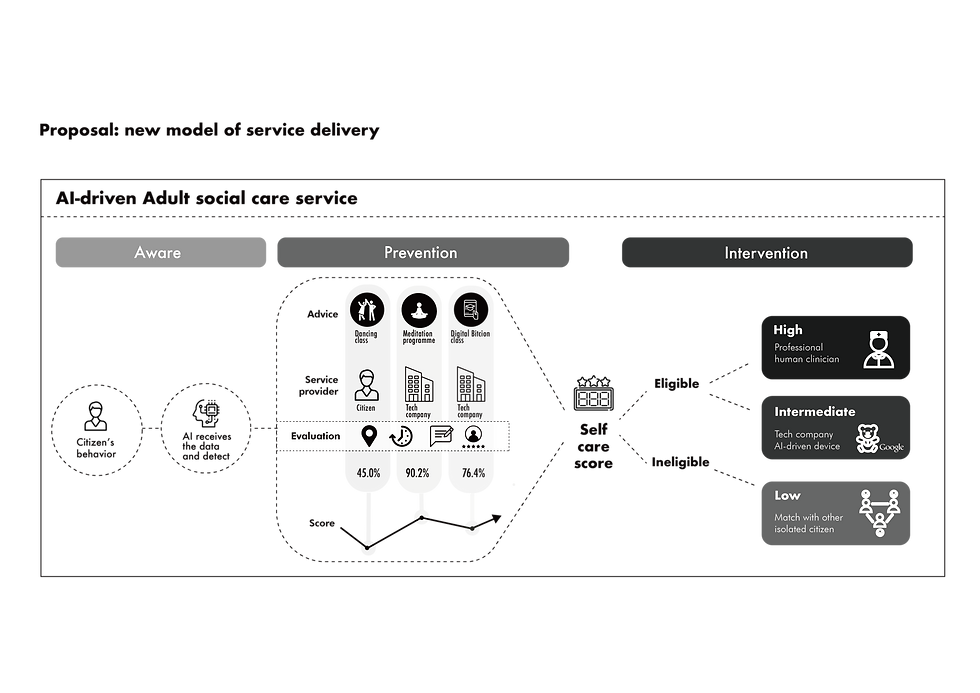
There are three stages in this AI-driven ‘public service-deliver model’ which are 'Aware, ‘Prevention’ and ‘Intervention’. We used the Adult social care as an example in our model to execute the service delivery. Citizens’ data is continually collected and analyzed by the government and tech company through artificial intelligence system. When the system perceive the citizen has the possibility in danger of poor mental health, the system will arrange the various activities that help the citizen maintain his/her wellbeing as prevention. Meanwhile, the system will generate the ranking score to evaluate people’s participation from prevention stage. Depends on the level of score, the citizen is able to access to different levels of public service in intervention when they need it
AI-driven decision-making process

This AI-driven decision-making rely on
a) Smart device that acquire people’s data
b) Cloud server that connect to physical network node and collect people’s data from them.
c) Machine learning and real-time analyzing system. The system will detect people’s condition and analyze people’s life styles and behaviors to generate tailored advices.
d) Matching system. With strong human resources and digital service’s database, the system will match the citizens to different services and other citizens who deliver the services.
e) Score system based on social credit structure.
Envision
Our model encourage citizens to engage more and put more efforts on the prevention process in order to get better quality of public service resources. It aimed to encourage citizens to take more responsibility for their own health and wellbeing.
The process of workshop
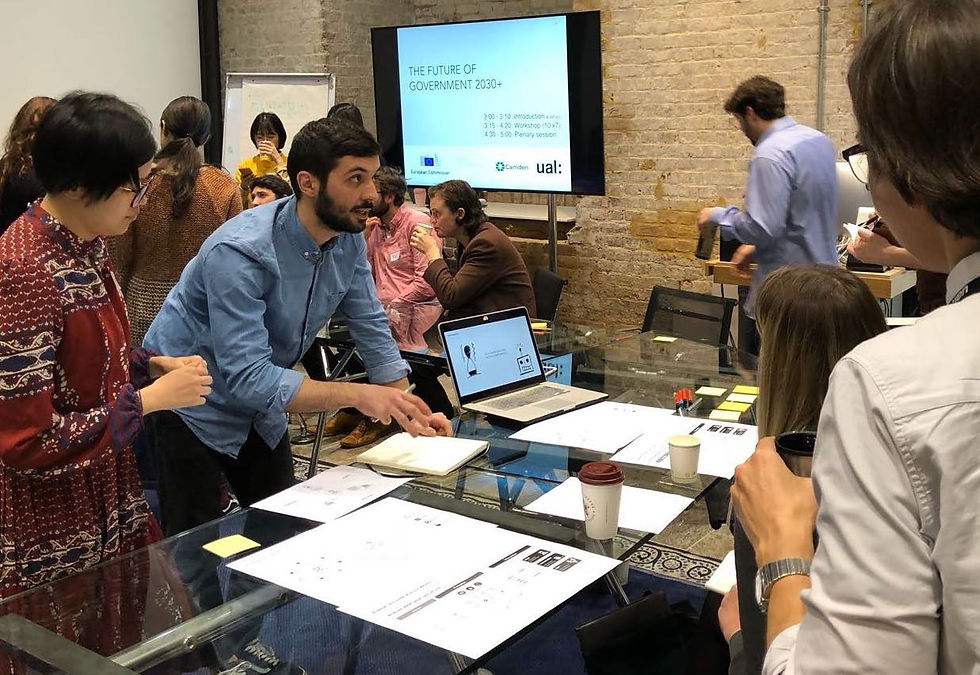
The co-creation workshop was about three hours in Central Saint Martin, University of the Arts London on 27 April 2018. The participants included the staff of Camden borough, professionals from NESTA, FutureGov, Cabinet Office - Policy Lab and academics from London universities.
According to our persona-Harry, we used the storytelling to present our concept, which helped our audience to imagine our scenario and questioned how to implement in a further way. Some people even had the direct emotional attachment instantly.
Overall, the majority of feedback was positive, and it helped us to develop our model in a more practical direction.
Feedback
AI targeting system
Our idea to use Artificial Intelligence to create tailored preventive recommendations was well received by the participants. They suggested us to use this system to connect the user to people in his network (‘When was the last time you texted David?’). A new way to promote positive behaviours and connect people with local environment. Some really interesting case studies emerged, for instance, the predictive analytic system used in Pennsylvania to fight children abuse.
Motivation in prevention
Isolated people will tend to be reluctant going out and meeting people. Engaging and motivating them won’t be easy. We need to explore further how the interaction between user and AI will be. Furthermore, the new system will create a lot of competition in prevention. On one side, the tech-companies might introduce in the market new preventive services (ambient location apps, virtual motivators, activity trackers, everything that can be used to keep the user connected, aware of his own condition and active). From a user point of view, the wish to keep the score high, might alter people’s behaviours. Attending an activity, being part of a community, and in general doing something for yourself might become something that you won’t do just for yourself, but to show people you are not a burden on the society, to give a good impression of you, to be matched with people active like you, to make sure you will be supported in case you might need it in the future.
What if you fail?
Some of the most frequent questions during the workshop was “Is there any possibility for the user to opt-out?”, “What happen if someone decides to ignore the recommendations?”, “What if I have a genetic or physical impediment is stopping me?”. We can describe our scenario as a “blaming culture”. If you fail, it’s your fault”. We need to consider that for someone the prevention system will work but for others no. It will be interesting to imagine some failing cases and speculate on the consequences.
It would be also worthy to see how the different social classes are affected in the same way. Rich people might be able to pay for services while for poor people the only chance might be follow the program.
During the workshop there was a long discussion about the intervention part. Some of the participants raised concerns about the kind of support the user should receive in case he/she eventually need Social Care services. To quickly recap, in our vision, if you support prevention you will get a more efficient support in case of need, while if you don’t, you will be just connected with people isolated like you. Some people suggested it should be the other way round: if you prevent enough you should just be connected with other people, whereas if you don’t do anything to prevent, the council should intervene and help you in a more sensitive way (paying a human specialist for example). Phoebe from London Borough of Camden reflected on this: “Is the main focus of the service rewarding good behaviours or mitigating the risk?”
Does this system have any tolerance for self fail user?
What happens if the user always has a low score with bad performance in prevention activities?
Cheating the system
Cheating the system is a point that arise more than once. What people will do to improve the score? Will they be moved by fear? But the question is “Will it be possible to cheat in a totally surveilled scenario?”
Economic aspect
How business works in the system across different stakeholders? How insurance is affected by this new model of service delivery?
If we consider that the currency might change and we might pay services with data, how this might affect the social care service delivery?
Our next step
After assembling the feedback from workshop, here are several challenges for following stage below:
The further research for AI targeting system
The implementation of AI is a vital role in our model. More research on the AI targeting system in the aware and prevention part is necessary.
Exploring different scenario of social isolation
What might become the obstacles for people to follow the prevention program? What if people has multi-condition?
How to focus on our final outcome
We had several controversial discussion in the intervention during the workshop, maybe we need to talk more about that with Camden participants.The further discussion will be essential to decide if we just focus on one phase between prevention and intervention. How to assure the people have appropriate motivation is another uncertainty for this group. It is necessary to find case studies of reward systems to motivate people in prevention.